In our previous article, we discussed what data preparation is and why it is so important for organizations. Today, we will look at the phases carried out within this process.
The reality is that there is no single "correct" way to approach the data preparation workflow, as each industry, project, or situation may require slightly different approaches. It's like with lentils, everyone cooks them differently: your grandmother one way, your mother another, you another, and in a restaurant, a completely different way. The point is that they always taste exquisite.
In this article, we have attempted to group the most recurrent tasks that occur in the data preparation process into six fundamental phases. Let's see them!
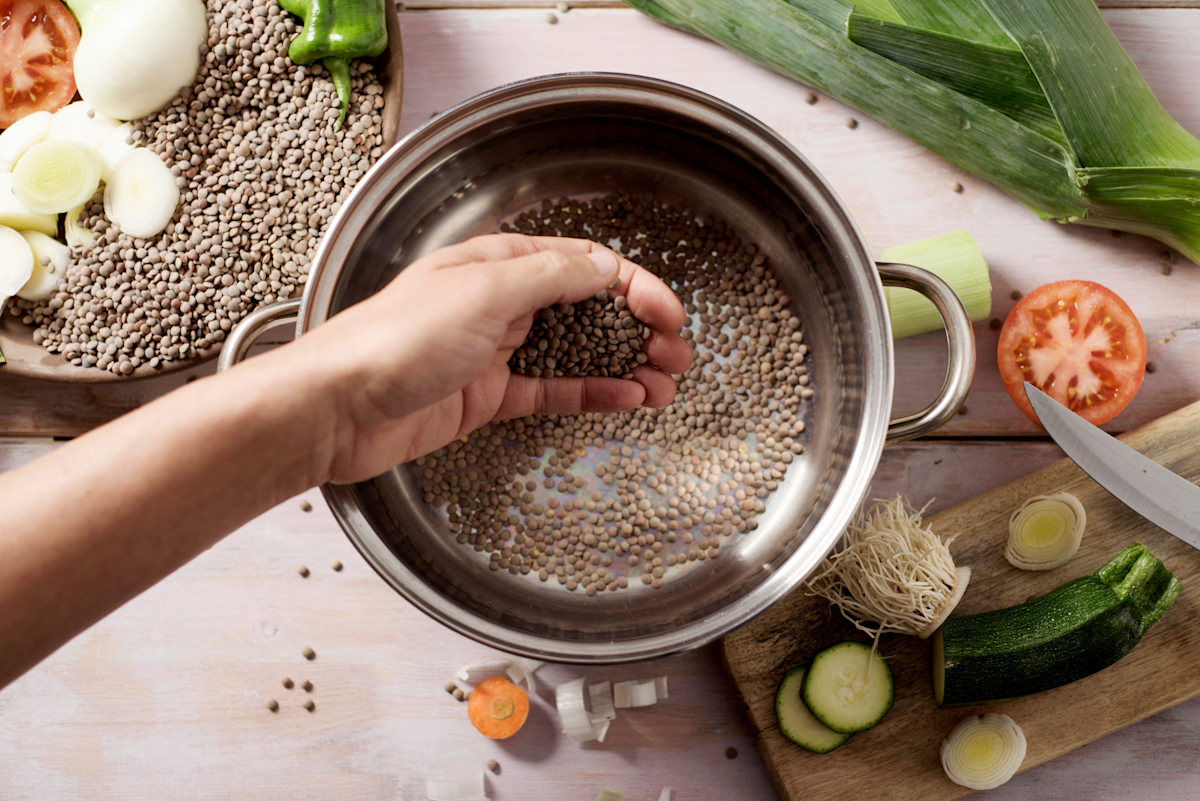
1. Collection
The first step of any data project is to gather the necessary data. Thus, in this phase, data is collected from various sources, whether databases, files, external sources, sensors, historical records, social networks, cookies, etc. The most important thing is that the sources are reliable and relevant to ensure the quality and relevance of the collected data.
2. Exploration
The second stage is to immerse yourself in the data and explore it in detail. In this phase, the main objective is not to conduct exhaustive analysis or seek correlations, but rather to detect possible errors that may have slipped through. It is essential to identify empty fields, verify data formats, and ensure they have the proper structure. A useful way to do this is through quick visualizations, as they can provide an immediate insight into the quality of the data.
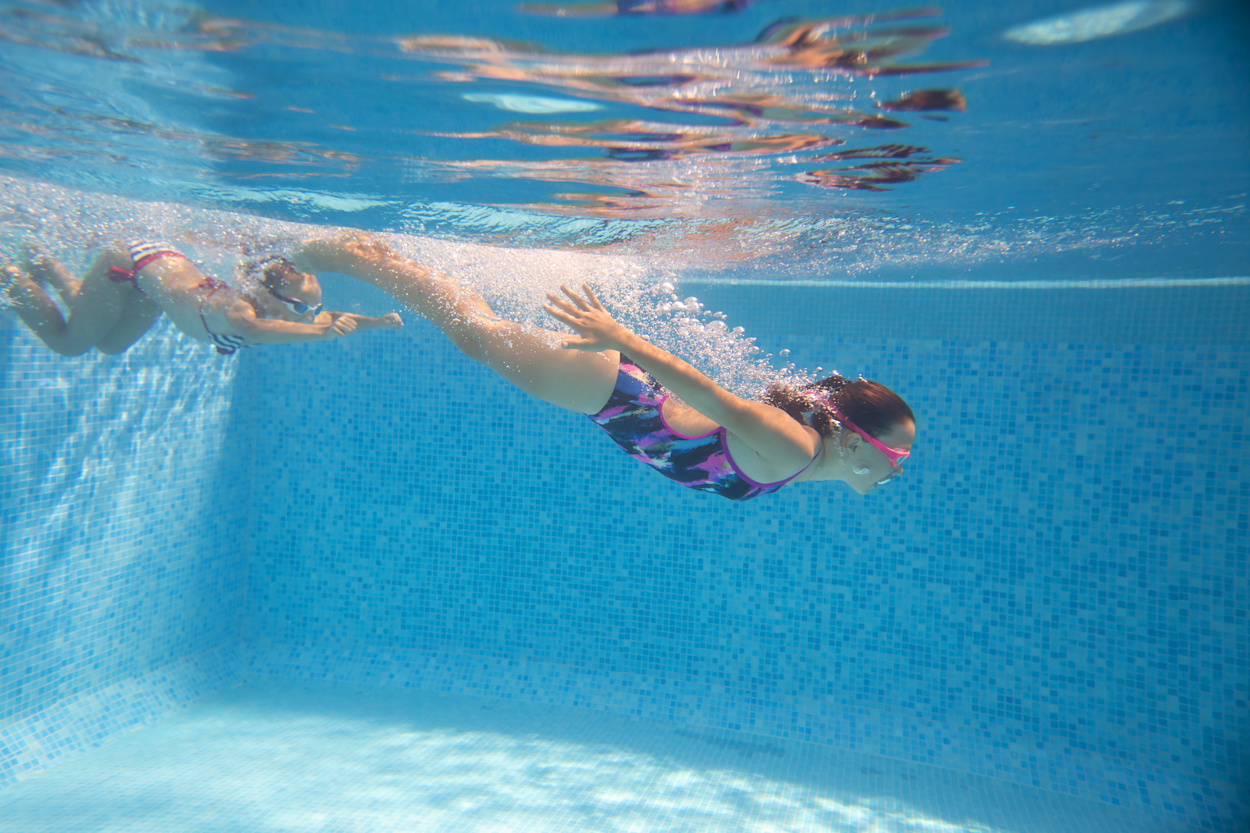
3. Cleaning
It is the most important phase, where you have to remove impurities and correct errors present in the data that you have witnessed during the exploration phase. Tasks such as handling missing values, removing duplicates or outliers, suppressing missing data, hiding confidential or sensitive information, correcting input errors, etc., are performed.
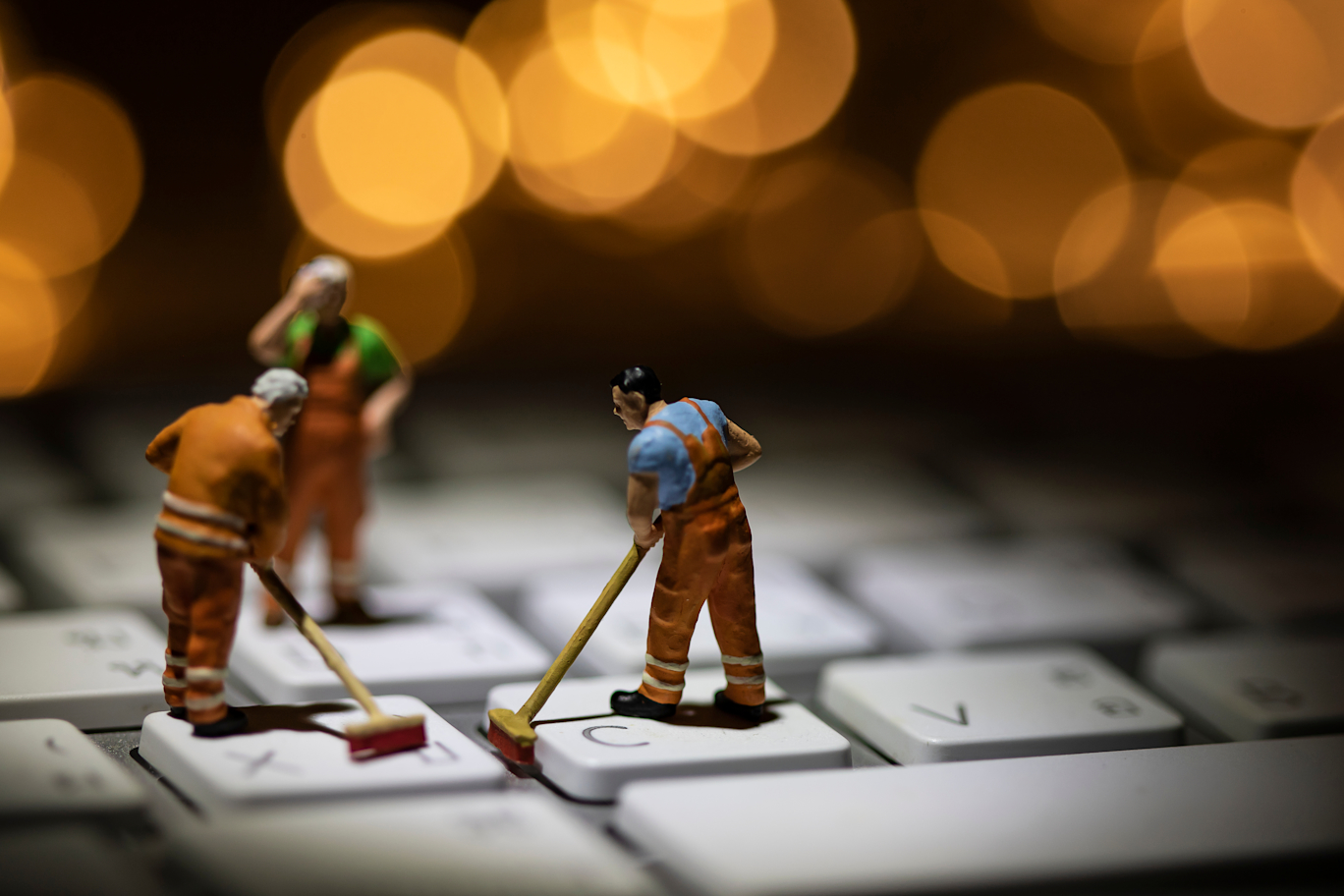
Integration
If the data comes from multiple sources, it is necessary to combine them into a single coherent repository. Integration may involve resolving inconsistencies in formats, merging duplicate records, and establishing clear relationships between datasets.
Transformation and Enhancement
This stage involves converting data into a suitable form for analysis and modeling. Some may be ready for analysis, while others may seem like a language similar to Chinese. Therefore, they must be transformed to ensure they can answer the questions you want to ask them. This phase may include variable normalization, data aggregation (such as external data, sales data, for enhancement), creation of new derived features, and application of calculations or mathematical functions to obtain more meaningful information.
Organization
In this step, the prepared data is structured in a format that allows easy access and querying. This may involve creating tables, databases, or specific structures according to project requirements or tools used.
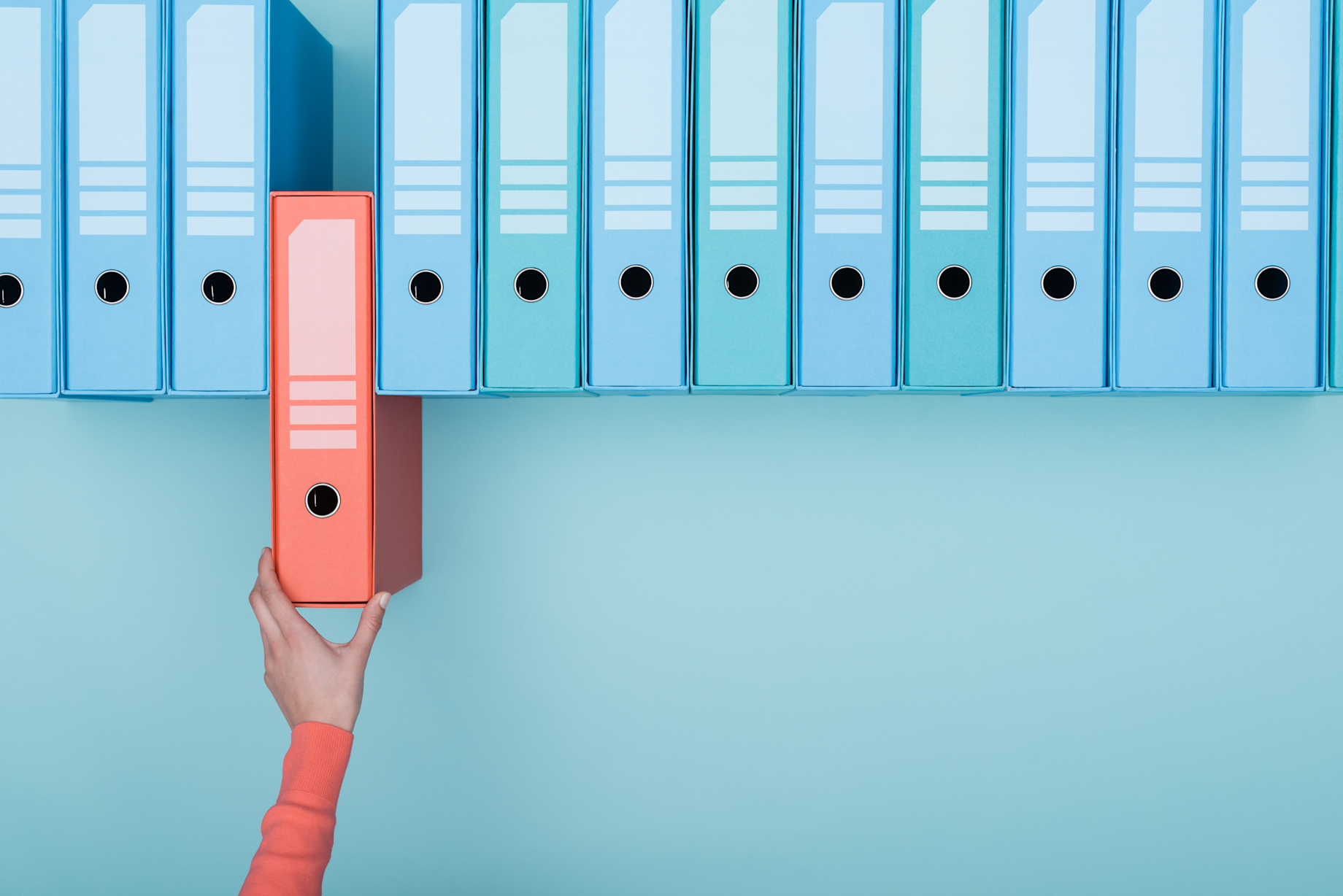
But beware, data preparation is not a one-time process. It is a constant commitment to the quality and relevance of information. As projects evolve, organizational needs, and data sources, data preparation must also adapt. It is a continuous journey of refinement!
Each of these fundamental phases we have explored plays a critical role in this ongoing process, helping to ensure that data remains a valuable and relevant tool over time. So, in a world where decision-making is based on data, the ability to prepare them correctly is a real competitive advantage for any organization seeking excellence in data analysis and decision-making.