Every organization that wants to take solid steps using its data as a strategic asset must employ standard techniques to carry out data lineage within its organization. These techniques include:
Pattern-Based Data Lineage
As the name suggests, this technique investigates lineage by sweeping and searching for significant patterns in metadata. It evaluates tables, business reports, and columns within disparate datasets for similarities indicative of redundancy. By finding highly similar columns with corresponding values, it links them in the data lineage graph to account for data at various stages of its lifecycle.
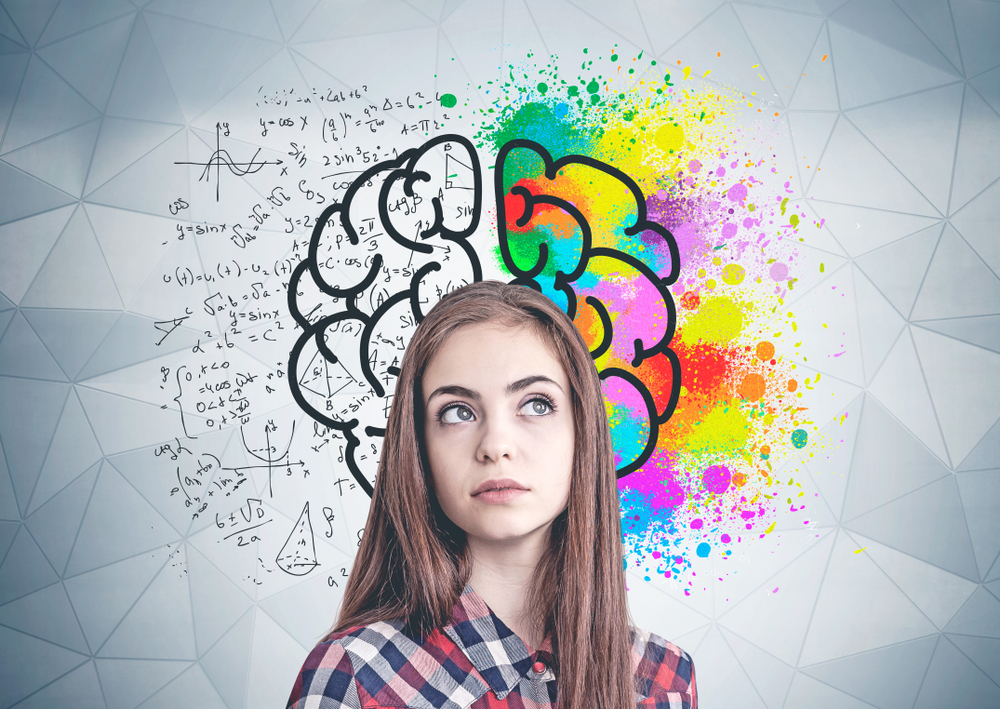
This technique does not vary with database technology and can do the job regardless of algorithms or technological advances. However, it cannot access data processing logic if embedded in program code. It can only explore metadata that is human-readable.
Analysis-Based Data Lineage
This is a highly advanced method for performing data lineage, which reverse engineers data transformation logic to achieve end-to-end data tracing. It requires an understanding of every programming language and tool involved in data transformation or alteration, making it extremely deep and comprehensive.
Data Tagging
Data tagging is most effective in closed data systems, where there is consistency in the tool used to transform or move data. Data tagging operates on the premise that a transformation tool or engine places an identifiable mark (a tag) on the data, which tracks the data from beginning to end.
Self-Contained Data Lineage
As the name suggests, this data lineage format works best within a self-contained data system or environment that includes processing logic, master data management, and storage. Such controlled environments include a data lake, which is a repository of all data at all stages of its life, facilitating access to data, albeit within the confines of the self-contained system.
Combine Data Lineage with Other Data Practices
Data lineage is a step in a robust data process. An organization needs a range of automated techniques, software, and practices to ensure good data management. Each of these practices intertwines with data lineage to form a solid framework.
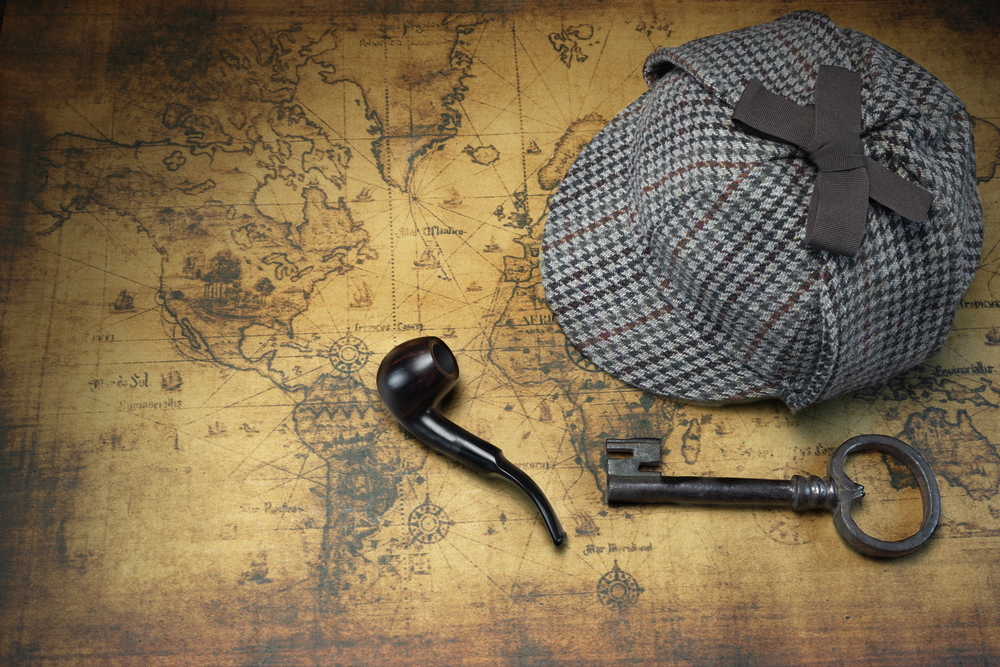
For example, data classification is used to find data that is confidential, critical, or requires some level of compliance. Data classification works with data lineage by investigating the data lifecycle, finding integrity or security issues, and helping to resolve them.
Sort Out Your Data Basics
Your data situation will never improve unless you take steps to address it. The amount of data collected, processing speed, and data legislation will only increase. You need to find a data management solution now. Alteryx has the answer, with powerful built-in data analysis and management tools.
If you leave your data unprotected, disorganized, and without lineage tracking, you are leaving your organization open to errors, fines, and loss of customer trust. With Alteryx, you'll enjoy a solution that helps you centralize and catalog data, streamline discovery, foster collaboration and data sharing, and understand the reliability of data assets.